December 2021 Issue Index
Geology tools solve engineering project
Maptek set a challenge to geologists to apply new software tools to a geology related application of their choice, with an emphasis on innovation.
Machine learning is new, exciting technology and one that is proving successful in generating rapid models using large quantities of data. One of the biggest benefits of Maptek DomainMCF is that it leaves more time for geological analysis and evaluation.
The Maptek Geology Challenge offered participants a chance to experience the benefits first hand by providing access to software tools for applying to a geology related project, with an emphasis on innovation.
Richard Jackson, Senior Technical Lead, Maptek and organiser of the global challenge said he was thrilled with the effort from all participants.
‘The winning entries were strong examples of well-defined problems that were difficult to solve with traditional methods, highlighting the benefit of using machine learning to act on multiple data types to create a geological model,’ Jackson said.
Winner of the challenge, which had to be completed over one week, was Henry Dillon, a Senior Geologist with global consultants, Golder, a member of WSP.
Dillon, who works in Christchurch, New Zealand applied Maptek Vulcan, Vulcan GeologyCore, Vulcan Data Analyser and DomainMCF to model complex shallow surface geology beneath a proposed engineering structure.
‘I had been wanting to try DomainMCF for some time, since I first started seeing it used and being talked about,’ Dillon said.
‘Our key problem was how to use all the data in the boreholes to model all the geologies and still get the low and high density sands in the right places throughout those drillholes,’ he continued.
Dillon’s answer was to assign and use numeric values, and combine the data with soil behaviour types and shear wave velocities to control the geological model.
DomainMCF handled complicated interfingering of sandy and silty materials and modelled sand–gravel interactions, generating the complex lithological interactions expected from braided river systems.
Speed is a known benefit of DomainMCF and proved to be the case for the Christchurch study.
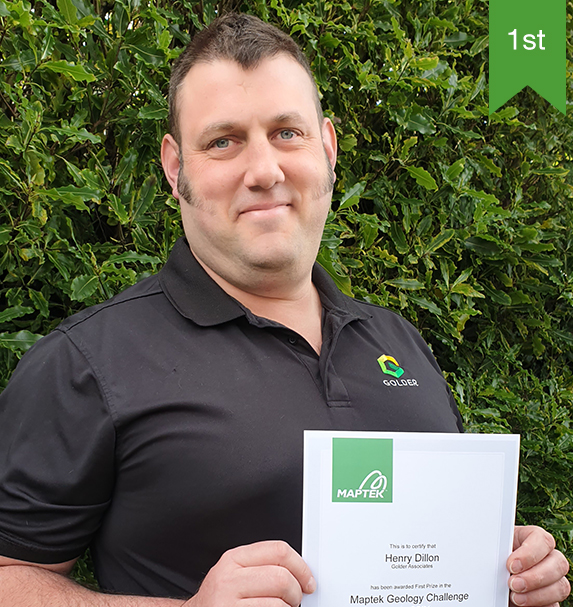
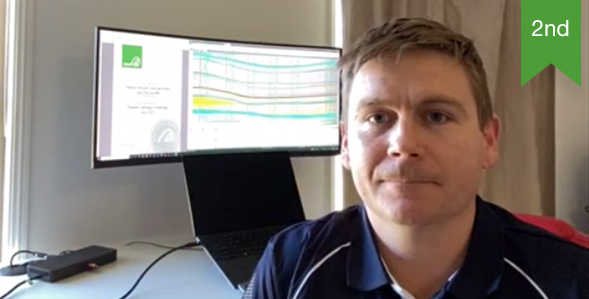
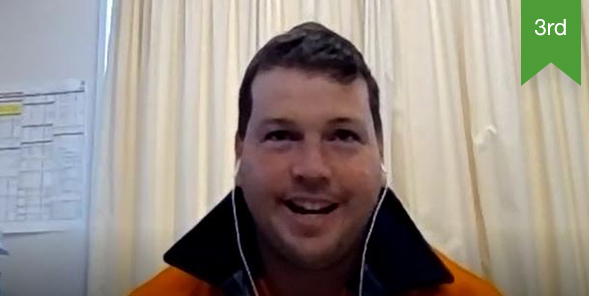
‘We were able to construct a reasonable lithological model from drillhole and cone penetrometer test data for an area of known geological complexity. We sent 193,000 data points to DomainMCF and received our model after 13 minutes and 6 seconds!’ Dillon commented.
As well as the innovative approach to geotechnical assessment of performance and design for the foundation of a future structure, Dillon provided invaluable feedback for improving the integrated modelling solution for all users.
‘DomainMCF has targeted the mining industry, but many other industries find traditional modelling processes to be equally time consuming – we can spread the benefit,’ Dillon commented.
Second place in the competition was awarded to a team from Anglo American led by Reece Stewart, for their innovative approach to overburden definition modelling, with third place going to Matt Green, Evolution Mining, who compared implicit modelling to DomainMCF for interpreting complicated geological structures.
‘Submissions displayed a range of novel techniques and applications that will contribute to innovation in our industry,’ Jackson concluded.
Maptek anticipates running another challenge in 2022 and looks forward to ongoing industry collaboration to simplify modelling processes and inspire geologists to find additional applications for the machine learning technology.
- Henry Dillon, Senior Geologist with global consultants, Golder, wins the inaugural Maptek Geology Challenge
- The winning project used Maptek software tools to model complex shallow surface geology beneath a proposed engineering structure
- Dillon used DomainMCF to quickly create a model from complex data (193,000 data points in 13 minutes, 6 seconds)