Reaching the rapid modelling goal
Maptek has developed a new solution for evaluating projects, progressing from database to resource report in 30 minutes.
Establishing a conventional ore resource report for a typical deposit can absorb weeks or months of geological effort. In response to the demand for faster results, Maptek has developed a new solution which unlocks huge potential for evaluating prospective projects.
Current reality
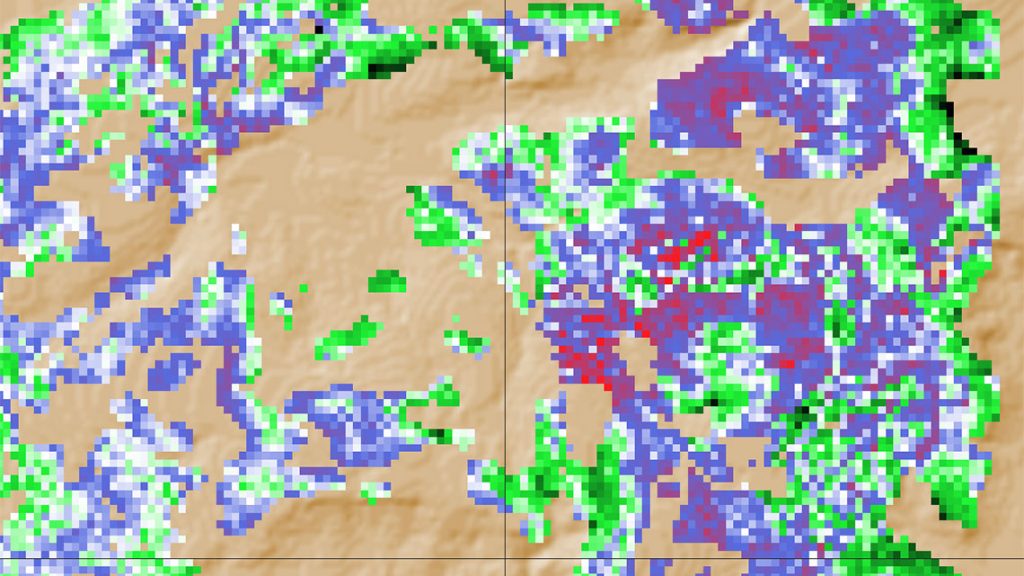
After database validation and construction of block model geometry, the next phase involves interpolating grade into individual blocks. Commonly used grade estimation techniques include inverse distance, kriging (multiple variants) and conditional simulation (also with a multitude of flavours). These have been developed and redeveloped, chasing the goal of better prediction of in-situ grades within ore deposits.
‘Mathematical techniques on their own cannot deliver realistic grade estimations,’ said Product Manager Steve Sullivan. ‘The geologist provides an intuitive understanding of the deposit geometry, which is key to guiding algorithms to the ultimate goals.’
Estimation algorithms have been deployed across industry in many software platforms. However, setting up an estimation for a single variable into a single geological domain using the inverse distance technique entails up to 190 decisions.
Likewise, applying ordinary kriging to a single variable/domain takes up to 170 decisions in preparation work alone, to determine appropriate parameter settings using variography and kriging neighbourhood analysis, followed by a further potential 220 decisions to carry out the kriging estimate.
Deposits rarely have a single commodity of economic interest within a single uniform geology. For example, one Maptek customer has approximately 900 variable/geological domain combinations. Applying kriging to estimation of the entire deposit requires about 35,000 decisions.
Little wonder that establishing a conventional ore resource report involves weeks or months of geological effort. Time constraints often do not allow the competent person responsible for the resource reporting process to validate each and every decision.
Future proofing
Through consultation with key customers, extensive R&D and testing with real datasets, Maptek has now developed a new solution to expedite resource modelling and reporting.
The deep learning approach, powered by Maptek’s machine learning engine, has already proven beneficial to the mining operation that partnered the development.
The geological database is uploaded into the deep learning system with several selected parameters. Data analysis leads to automated assignment of estimation parameter settings, followed by geological domain interpretation, grade interpolation and uncertainty analysis.
The results are downloaded in standard Maptek block model format for resource reporting and collaboration with other users of the geological resource model, such as geotechnical, mine planning and mine scheduling engineers.
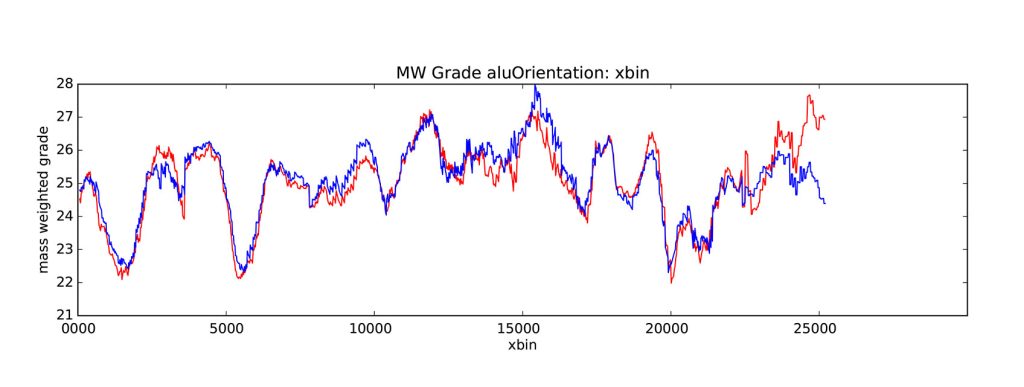
Benefits
The deep learning process models multivariable/multidomain data simultaneously. Input data is validated prior to modelling and results are validated with standard charting techniques.
From geological database to resource report takes about 30 minutes, compared with weeks or months for conventional processes.
This is a truly rapid modelling technique. Resource modelling using the Maptek machine learning engine will be available in 2019.
Contact solutions@maptek.com.au