Modelling marble reserves
Applying machine learning to model marble reserves resulted in faster results and more uniform quality classifications to guide extraction.
Marble quarrying is an important industry in northeastern Greece, and standard reserve estimation and modelling techniques present several challenges.
Machine learning systems take advantage of the computing power of personal, embedded and cloud computing systems to rapidly build models of real processes.
Marble reserves are based on marble quality categories, unique to each deposit or quarry. The categories represent visual and physical characteristics such as colour, texture and fractures. Classification is typically conducted by experienced personnel on samples that are much smaller than the blocks that are mined.
Maptek DomainMCF was applied to marble classification at the Volakas Quarry, owned by Iktinos Hellas SA, and compared to a previous Maptek Vulcan study.
The following parameters were identified and used to characterise Volakas marble:
- Lithology – Dolomite or calcite
- Type – Flower or diagonal veins
- Background – Visible defects
- Tectonic – Discontinuities/sq m
Iktinos Hellas has been using Vulcan since 2014, employing an inverse distance method on the block model. Block dimensions are configured based on the volumes extracted from the quarry.
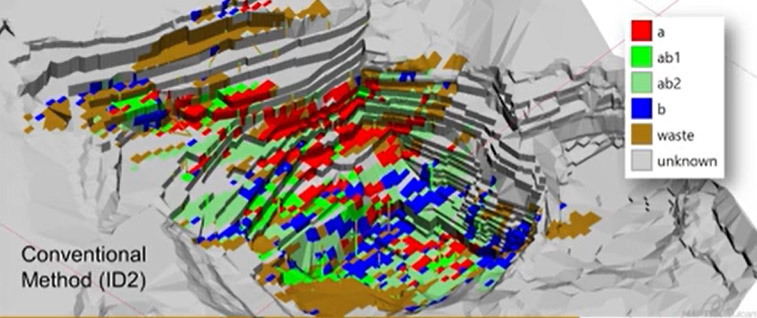
Samples are seeded around each block using search ellipsoids oriented according to geological features. A block model script is run to assign a final marble classification that consolidates the interpolated indicator field value.
DomainMCF models the spatial distribution of the marble quality characterisation parameters by sampling a discrete set of domain values. The data for the Volakas study consisted of 95 drillholes and quarry face analyses, giving a total of 3570 one-metre samples.
Sample data was composited in separate files for each of the marble quality parameters and then run in DomainMCF. A block model definition file was applied to control the application area, also limited using an upper and lower surface.
DomainMCF is fast and simple to set up and run, and operates directly on drillhole and other data.
Processing 3750 samples and 2 million blocks took less than 2 minutes to complete.
Comparing the marble quality classification produced by DomainMCF to the conventional method, it was clear that the machine learning classifications appear more uniform for the blocks included in both methods.
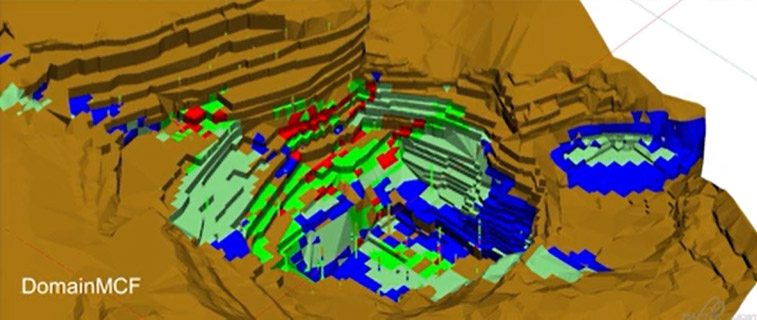
The machine learning engine also requires no structural analysis of the categorical parameters and provides a measure of uncertainty for predictions. This is useful for identifying areas where more sampling may be required or existing samples have higher variability, leading to less certainty.
Future studies include using anisotropy for predicting parameters, better understanding of confidence levels and how they can be associated with resource categories and investigating the influence of grade on domain predictions.
Dr Ioannis Kapageridis
Associate Professor
Department of Mineral Resources Engineering,
University of Western Macedonia
- Machine learning for domain modelling improved the speed and uniformity of marble classifications in this resource estimation study
- DomainMCF is fast and simple to set up and run, and operates directly on drillhole and other data, allowing models to be updated regularly
- The machine learning engine requires no structural analysis of the categorical marble parameters, further streamlining the process