March 2021 Issue Index
Harnessing deposit complexity
Machine learning techniques use all data sources for geological modelling, enhancing the understanding of complex deposits and improving decision making.
During development of Maptek DomainMCF, a review was undertaken of resource reports lodged to statutory bodies. This found geological models with over-smoothed lithology boundaries, many of which do not represent how geology appears in the field, pit or underground development drive.
The underlying data is often too complex or messy to be incorporated into the resource model and is thus a simplification of reality, to the detriment of the mining operation and its shareholders.
These approximations can be improved with new modelling techniques such as machine learning. However, with poor data or improper use of machine learning techniques, the conclusions can be just as misleading as with current techniques.
Complexity in deposit modelling is accentuated by at least six factors: data diversity, structural controls, chemistry, data volumes, process workflows and external constraints.
Data diversity
Models are built to represent the underlying geology for use in resource estimation, reporting, geotechnical studies, geometallurgical work and mine planning.
Relevant data can be sourced from a range of technologies such as geological logging, geochemistry, geophysics, geotechnical, hyperspectral, pXRF, photography and lidar.
Each source uses different data formats and provides differing levels of accuracy and relevance.
As well as merging data from different backgrounds, an environment for analysis, interpretation and modelling must be able to process the complex array of disparate data.
Structural controls
Many mineralised systems have been formed by controlling structures while others have been modified since emplacement by post-mineralised events such as folding, faulting and/or shearing, sometimes of multiple generations.
Understanding the structural framework is important in deposit modelling.
The sequence or hierarchy of complex events such as post-deposit faulting or cross cutting dyke emplacement will impact geotechnical competency and mine planning studies.
Chemistry
Mineral deposits are natural enrichments of elements or compounds of economic interest. Deposits can be simple in geometry but complex in mineralogy and chemical composition.
This complexity can exist in the economically important minerals where they are locked in refractory mineral species. And where deleterious elements are intimately entwined within the mineralisation their distribution also needs to be understood.
Data volumes
The increased availability and variety of sensors collecting data for geological modelling has led to a significantly greater volume of data.
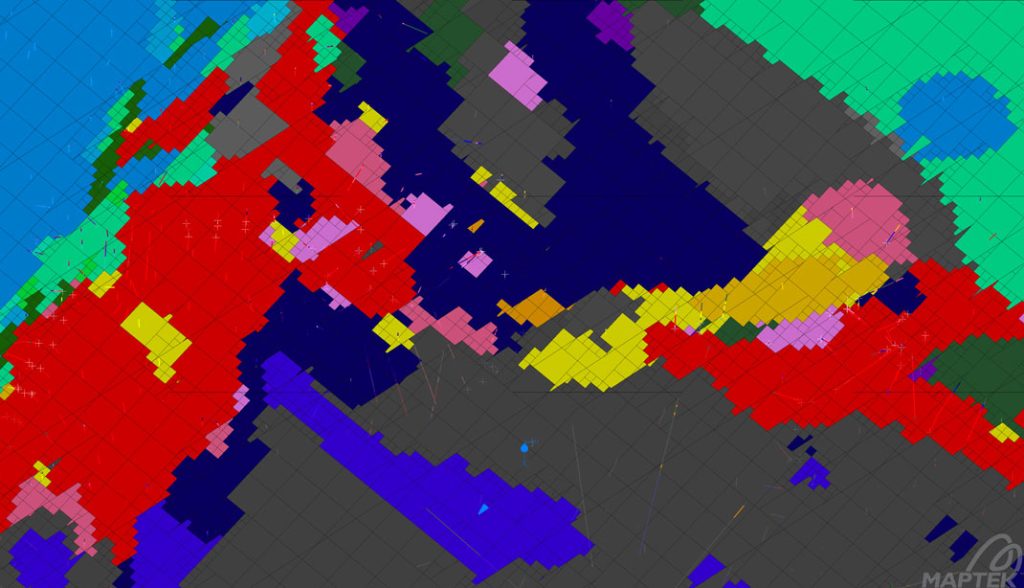
A decade ago, input data sizes may have been measured in megabytes or gigabytes; the current generation of core imagery from hyperspectral sensors can generate terabytes in minutes.
Managing the validation, integration and usefulness of this data is a significant challenge for operations and adds to the complexity matrix.
Process workflows
Many customers use a lot of different software to manage and process their geological data.
Having complex software interactions adds an overhead for IT application management and data flow, requiring validation that the process is not broken by software upgrades.
There is also increased potential for data transfer errors. Learning multiple new software interfaces each with their own method of operating can be confusing, so it requires more effort to induct new personnel into a team.
External constraints
In addition to technical constraints external complexities can impact the production of geological models.
Continuity of geological staff is a principal concern for many operations. Multigenerational mines require stewardship through the hiring/firing cycles that follow the highs and lows of commodity prices. Shorter-lived or remote operations using fly-in fly-out staff, can experience an inconsistent and distracted workforce.
It is important to maintain geological data integrity, regardless of who is collecting and recording it.
Case history
In a recent project for a deposit adjacent to an existing mine in a complex structural setting, more than 50 lithology codes had been reduced to six composite codes for modelling. This reduction of complexity, including ignoring any zones that had been logged as faults or shears, resulted in an overly simplified model.
Presenting the full richness of the data to DomainMCF resulted in a model with a complicated mixture of faults and shears that dislocated lithologies.
Use of all the geological information in the resultant resource model brought new light to understanding the deposit, providing a basis for more informed mine planning decisions.
Machine learning rarely offers a single ‘right’ outcome, rather a range of possible outcomes from the data provided. Does it provide meaningful information in an easily accessible way? Absolutely, and rapidly.
Use all the data, use DomainMCF.
- Poor data or improper use of machine learning techniques can lead to outcomes that can equally misrepresent reality as with current techniques
- Machine learning rarely offers a single ‘right’ outcome, rather a range of possible outcomes from the data provided
- DomainMCF uses the full richness of the geological information to provide better understanding of complex deposits