December 2022 Issue Index
Confident, controlled domaining
Maptek will introduce new audit controls and confidence measures for geologists, with a range of persistent model features taking the spotlight in DomainMCF in 2023.
As more customers around the world adopt the Maptek machine learning approach to domain modelling, the development cycle benefits from wide-ranging user feedback. The latest DomainMCF incorporates new functionality addressing real needs and allows it to be embedded in day to day operations for mine and resource geologists.
Keeping an audit trail for a predominantly manual geological modelling process is often difficult. Direct input from geologists is required for the manual editing of database codes, interpreting data on screen or adding controls to assist wireframe generation. Without a clear audit trail it is not possible to exactly replicate a process.
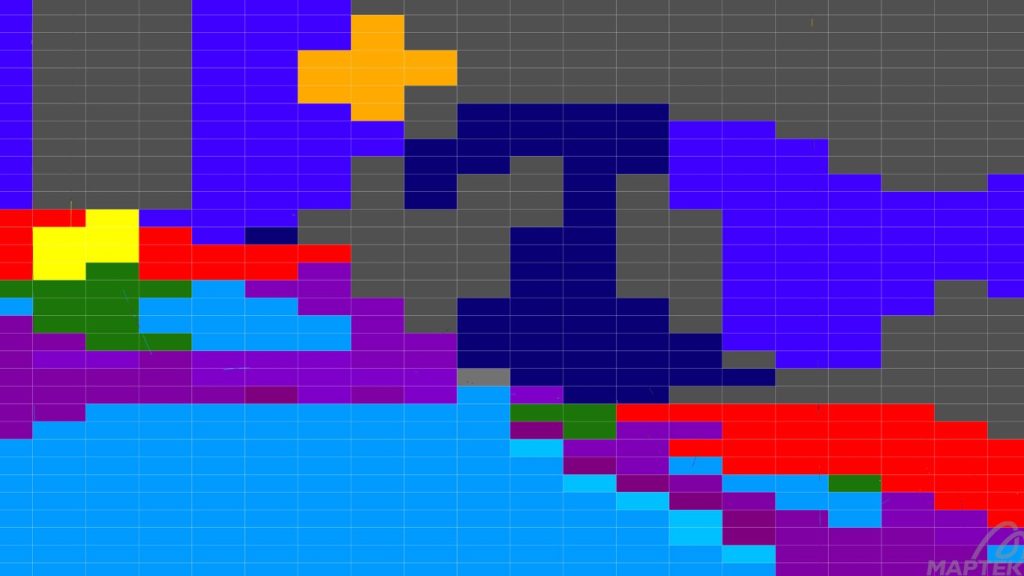
The latest version of DomainMCF will save a file that embeds the input data and machine learning modelling process for future use. The resultant machine file can be downloaded and archived as a persistent history of the geological model. As more data is added, a machine file can be saved to capture the incremental changes.
The persistent file allows users to experiment with block dimensions in resultant block models. Outputs with 20x20x20m blocks can be made rapidly and visually validated. If they pass all the checks, then smaller parent blocks or subblocks can be extracted from the same machine file, keeping the underlying model intact.
Generating outputs from the machine file reduces modelling time, compared with retraining the machine learning model in order to produce a different blocking size in the resultant block model. For example, geologists often build subblocked models to better reflect geological boundaries, whereas mine planners often require regular blocks for mine optimisation.
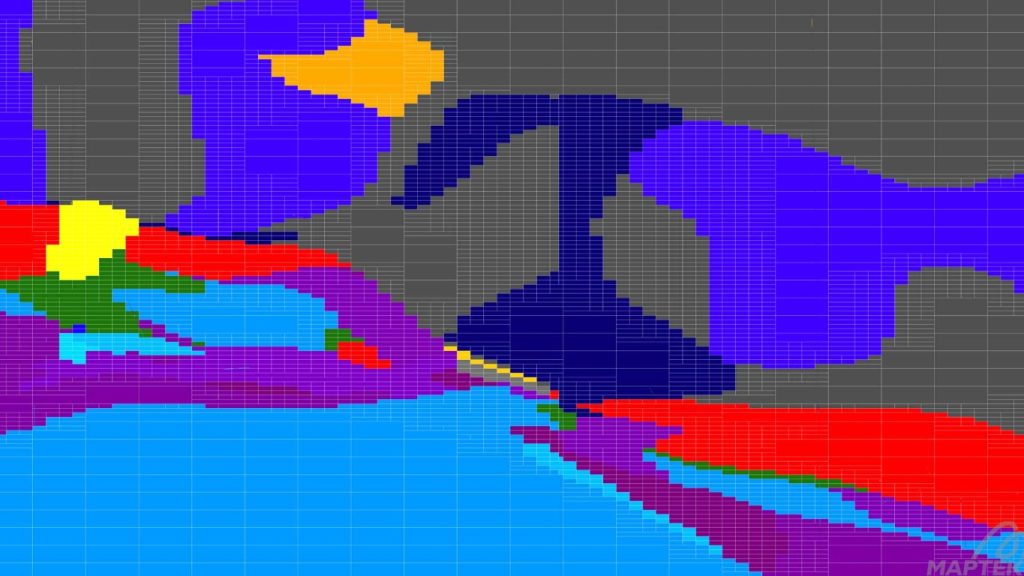
Now, both subblocked and regular models can be extracted from DomainMCF, ensuring consistent output from an auditable model file.
The 2023 release of DomainMCF will provide even greater user control over the modelling process.
Previously, DomainMCF showed the estimated run time for the modelling process and then provided a hard stop if it reached double the estimate. This was designed to protect users from unexpectedly draining their subscription account balance.
When the hard stop condition eventuated, even when the status bar indicated the modelling to be temptingly close to completion, the process was automatically cancelled and the elapsed compute time added back to the subscription balance.
Now customers can set their preference to override the 2x time limit and keep processing, and if they elect to do so, set an upper limit for the job duration.
In 2021 DomainMCF introduced geological domain uncertainty, a useful feature allowing geologists to view areas of the deposit where machine learning was not confident of predicting the correct domain.
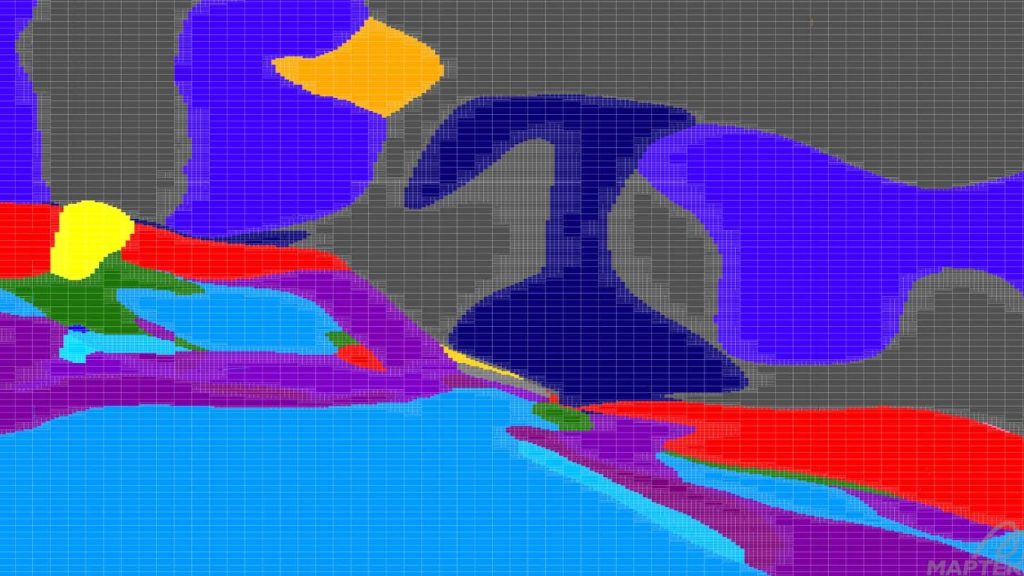
This typically occurs when there are several domains in close proximity without a clear geological hierarchy. However, when data is sparse on the margins of a model, geological boundary confidence is often deceptively high as there are no other domains nearby.
Now DomainMCF provides an option to assess sample point distance and save this as a separate variable in block model outputs. Sample point distance measures the distance from the block centroid to the nearest input sample point, and in combination with the domain boundary confidence provides a better measure of the certainty of the domain prediction.
The enhanced DomainMCF will be available for download from Maptek Account early in 2023.
- Geologists will welcome greater control over the machine learning modelling process and confidence in modelled outputs
- DomainMCF provides a persistent history of the geological model, capturing an audit process that reflects incremental changes
- New features in 2023 include flexibility to choose the block size for output models after machine learning has been completed