Accurate domain modelling
Making the most of machine learning for rapid creation of accurate models requires attention to data preparation, validation and management.
Machine learning algorithms can quickly analyse big, dense and complex data way beyond human capabilities. But algorithms aren’t wired to understand nuance or shorthand and make assumptions in the way people can.
Maptek DomainMCF uses machine learning to generate domain boundaries directly from drillhole sample data for rapid creation of resource models.
For decades, solutions such as Maptek Vulcan have given mining operations the tools to work with accurate, validated mining data. Steve Sullivan, who recently celebrated 25 years with Maptek, has been helping customers understand their geological data throughout that period.
Sullivan is part of the team behind the creation of DomainMCF and has tested it against more than 100 different deposits, covering historical datasets, operating mines and exploration projects.
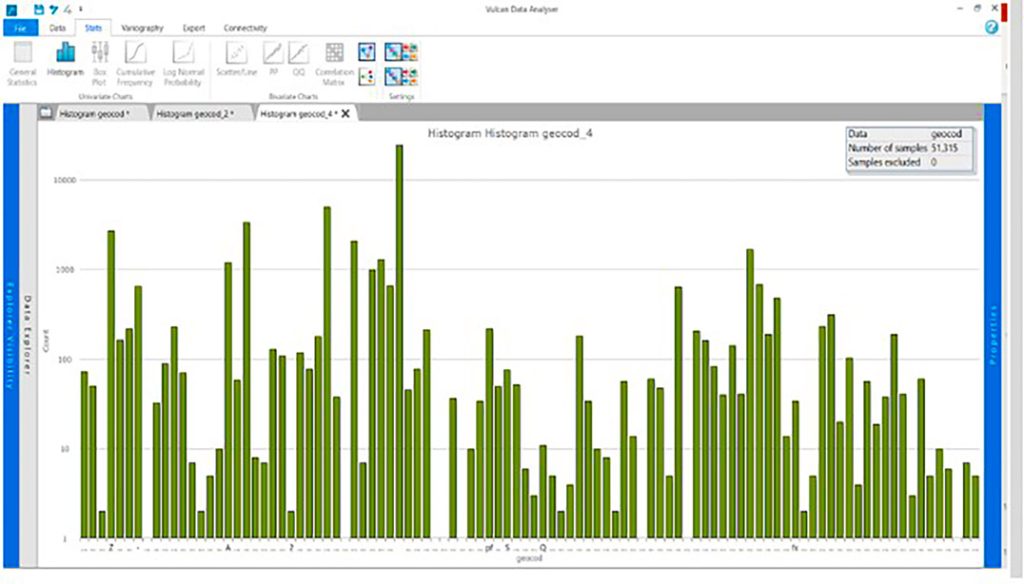
Sullivan said the results had proven DomainMCF’s ability to build accurate models in a fraction of the time of traditional methods but reiterated the importance of proper data preparation, validation and management.
‘It’s a slightly different way of thinking,’ he said. ‘When we work with other humans, we make decisions as we go.’
‘For example, when geologists see NL in a database they know, or infer that it means not logged – they can dismiss the information and move on. But a machine doesn’t know that – it may ‘think’ NL is a mineral or attribute code and does not know to ignore it.’
‘With machine learning we still need to teach the machines how to learn and give them context. When setting up a project for machine learning our decisions need to be taken beforehand to give the machine the best chance of identifying a meaningful answer.’
‘Our data needs to be squeaky clean. This can be challenging. Mines often have FIFO workers who use subtly different logging styles and may see different things as important, for example, vein percentage versus alteration assemblage.’
Processing the information is about setting up standards and applying them, Sullivan said.
‘The raw data doesn’t change, people’s interpretations of the data change.’
‘As every orebody is different, a separate set of standards need to be tailored for each deposit. These standards are based on a series of logical steps. If a certain condition is met, then this is the correct action to resolve the issue.’
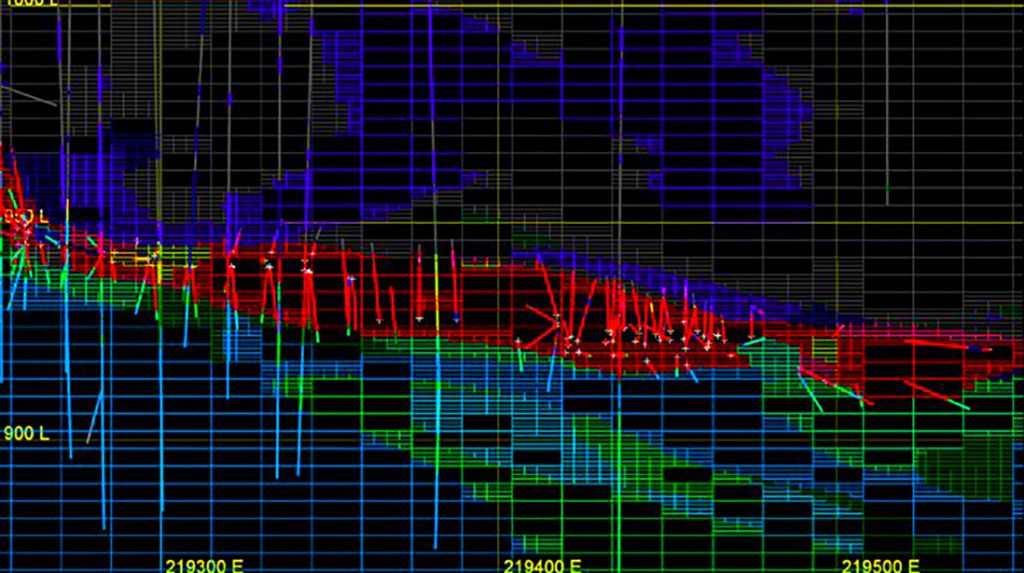
‘Database manipulation can be performed manually, each step at a time, or alternatively the standards can be applied through a series of processes or scripts. The advantage of the latter is that an audit trail is kept for reporting. Processes can be set up and run as a sequenced standardised workflow for repeatability.
‘This sets up the opportunity to leverage machine learning operations, enabling data science and IT teams to collaborate and increase the pace of model development and deployment.’
‘What at first may seem like an arduous task of preparing the data and standards is a valuable time investment as it unlocks the true power of DomainMCF,’ he said.
With machine learning, instead of modelling a resource deposit model once a year or every six months, users can generate a model every day as the incremental data comes in, because all the standards are there and it’s ready to go.
This is no longer a static model of an operation, it is a live, dynamic model where engineers and geologists are making decisions using the latest data.
The importance of being able to make decisions based on current information is evident when the metals prices are erratic, as they are right now.
- DomainMCF can build accurate models in a fraction of the time of traditional methods
- Proper data preparation, validation and management is of critical importance
- A live, dynamic model allows engineers and geologists to make decisions based on the current information