Machine learning case study
A new Maptek geological domain modelling process employing machine learning has delivered accurate shapes and volumes in much shorter time.
The Lisheen Mine, located in the Irish midlands in County Tipperary was featured in Maptek Forge in December 2001. The carbonate hosted zinc-lead mine was in full operation at the time using Maptek Vulcan software for underground survey, geological modelling, variography, resource estimation and mine planning.
The mine came into production in September 1999 and produced an average of approximately 160,000 tonnes per annum of contained zinc in concentrate and 25,000 tonnes per annum of contained lead in concentrate.
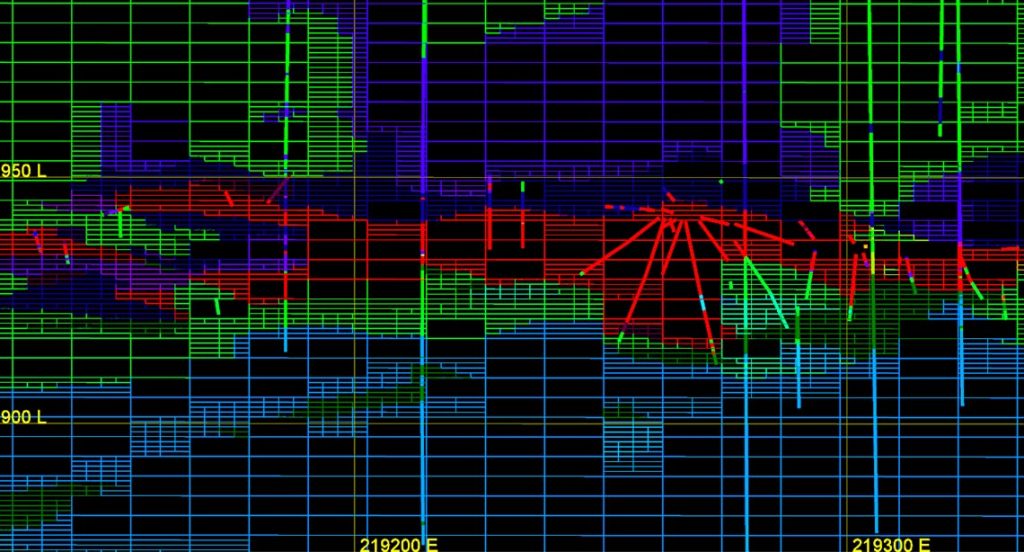
The mine was exhausted of ore in 2015 and this retrospective compares the geological modelling used during mining, with modelling using the new generation Maptek machine learning service.
The Lisheen mine lease area includes a total of 2700 surface and 4670 underground drillholes. Drilling was predominantly diamond drill core and was logged in detail by mine geologists. Rock types were classified into 50 different codes during logging and these were used for subsequent domain modelling.
It is not recorded how long it took to generate the wireframes to represent these geological codes. It would be safe to assume that many weeks were required to model the complex interaction of some of these units.
Maptek has developed a new geological domain modelling process using machine learning, which is especially suited to large volumes of data. The Lisheen mine lease data was entered into the machine learning engine for processing along with dimensions for the resultant block model, into which domains are directly written.
No other geological interpretations, aside from the rock codes in the drill base were used for processing. A parent block size of 12x12x4m was used with subcell sizes down to 3x3x1m for the subhorizontal ore bodies.
After 50 minutes processing, the resource block model was complete, flagged with domains generated for all 50 rock codes.
The recorded difference in the ore volumes between methods was only 4%, and the new approach was much faster.
The block model was validated visually using cross sections and long sections comparing drill codes and the block geology codes. These compared very favourably.
Following the comparison of the machine learning domains with the original drill codes, a check against the manual wireframes generated during mine operation was conducted. This also showed a very close match.
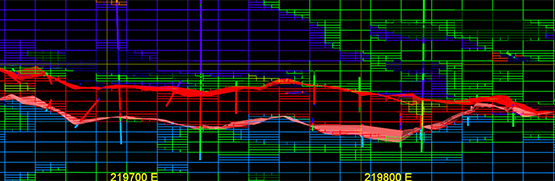
A volumetric comparison was then made between the mine resource model using manual wireframes to flag geological domains and the model built using machine learning. In summary there was only 4% difference in the ore volumes between methods, which is a very favourable comparison.
The machine learning model built from the interpreted codes in the drillhole database, without further geological intervention, has been able to replicate accurately the geological domain shapes and volumes.
Acknowledgment to Minerals Ireland for access to the Lisheen mine data.
- Embracing new technologies such as digital transformation and automation is a proven way to speed up the strategic planning cycle.
- Maptek machine learning model correlates geological database codes directly into a 3D block model, and then uses resulting domain codes to constrain grade estimation.
- A resource block model was complete, flagged with domains generated for all 50 rock codes after just 50 minutes processing time.